RFS: Machine learning to simulate the physical world
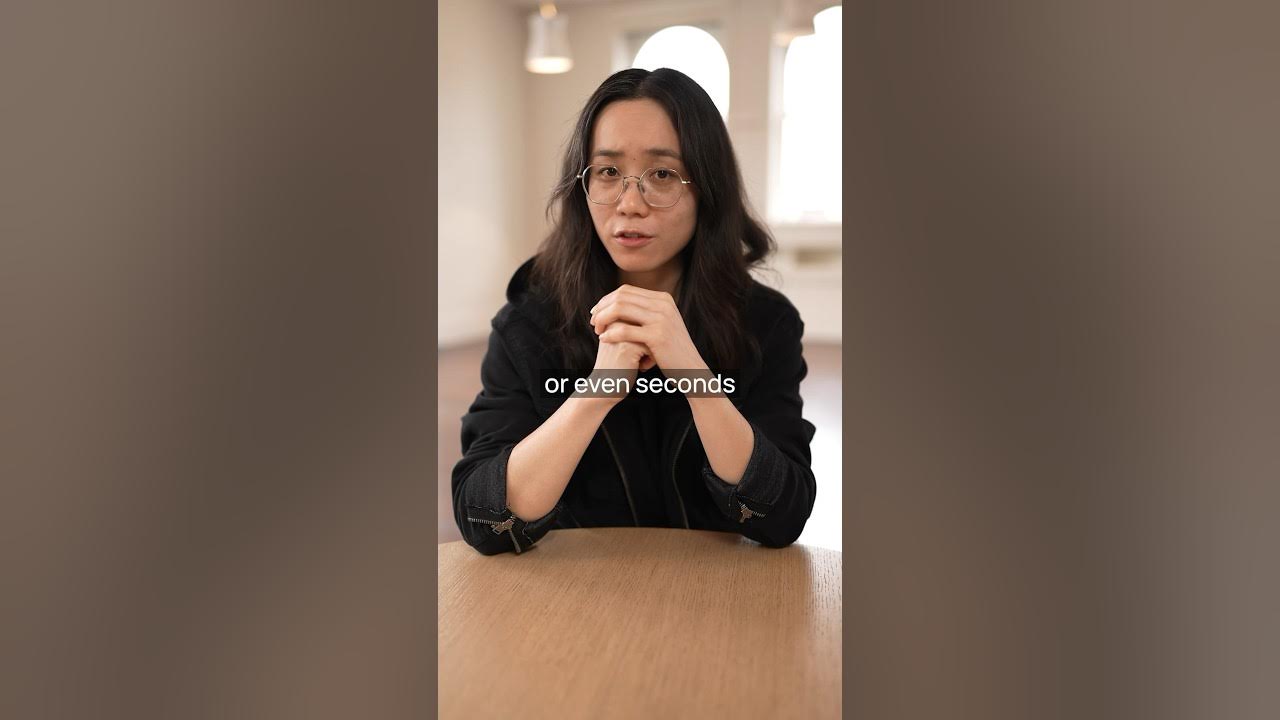
Table of Contents
- Introduction
- Machine Learning in Physical Simulation
- Applications of Machine Learning in Physical Simulation
- Challenges and Future Directions
- Conclusion
1. Introduction
The field of physical simulation involves creating computer models that mimic the behavior of physical systems. Machine learning techniques are increasingly being employed to enhance the accuracy and efficiency of these simulations. This integration of machine learning with physical simulation has opened up new possibilities for researchers and engineers to better understand and predict the behavior of complex systems.
2. Machine Learning in Physical Simulation
Machine learning algorithms are used in physical simulation to improve the accuracy of models and reduce the computational cost of simulations. These algorithms can learn from data to make predictions, optimize parameters, and even discover new physical relationships within the system. Examples of machine learning techniques used in physical simulation include neural networks, reinforcement learning, and Gaussian processes.
3. Applications of Machine Learning in Physical Simulation
Machine learning in physical simulation has a wide range of applications across various fields. In physics, it can be used to model complex systems such as fluid dynamics, quantum mechanics, and cosmology. In engineering, machine learning can help optimize the design of structures, vehicles, and materials. Other applications include climate modeling, robotics, and computer graphics.
4. Challenges and Future Directions
While machine learning has shown promise in enhancing physical simulation, there are challenges that need to be addressed. These include the need for large datasets, interpretability of results, and the incorporation of domain knowledge into machine learning models. Future directions in this field involve developing more efficient algorithms, improving model interpretability, and exploring interdisciplinary collaborations.
5. Conclusion
Machine learning is revolutionizing the field of physical simulation by improving the accuracy, efficiency, and scalability of models. By integrating machine learning techniques with physical simulation, researchers can gain deeper insights into complex systems and make better predictions. As this field continues to evolve, it holds great promise for advancing scientific discovery and technological innovation.