UniPi: Learning universal policies via text-guided video generation
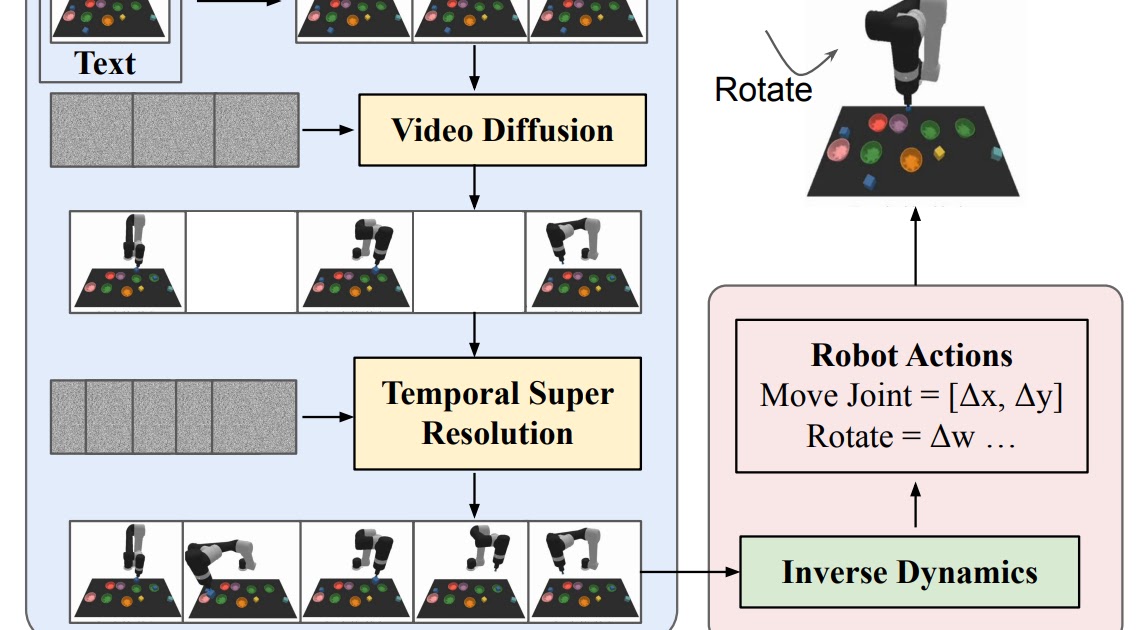
UniPi
UniPi is a learning model that uses text-guided video generation to construct agents capable of completing different decision-making tasks across diverse environments. It uses a novel video generator to generate video snippets showing an agent's trajectory in achieving a given task. UniPi allows the use of language and video as a universal control interface for generalizing to novel goals and tasks across diverse environments.
Video Policies Generated by UniPi
UniPi generates videos at a coarse level by sparsely sampling videos of desired agent behavior along the time axis. Given an input observation and text instruction, a set of intermediate key images representing agent behavior is planned, which is then refined into plans in the underlying state and action spaces. The inverse dynamics model synthesizes image frames and generates a control action sequence that predicts the corresponding future actions.
Capabilities and Evaluation of UniPi
UniPi has been evaluated by measuring the task success rate on novel language-based goals. It generalizes well to both seen and novel combinations of language prompts, compared to other baselines, such as Transformer BC, Trajectory Transformer, and Diffuser. UniPi is also capable of synthesize a diverse set of behaviors that satisfy unseen language tasks.
Multi-Environment Transfer
UniPi has been tested on novel tasks not seen during training, and it shows a high task success rate. It is capable of synthesizing plans for different new test tasks in the multitask setting.
Real World Transfer
UniPi is capable of generating videos given unseen real images for a different task. In contrast, a model trained from scratch incorrectly generates plans for different tasks. UniPi's video generation quality has been evaluated using the Fréchet Inception Distance and Fréchet Video Distance metrics.
UniPi is a promising step towards applying internet-scale foundation models to multi-environment and multi-embodiment settings. There is much potential for future research in this area.