Using Synthetic Data to Address Novel Viewpoints for Autonomous Vehicle Perception
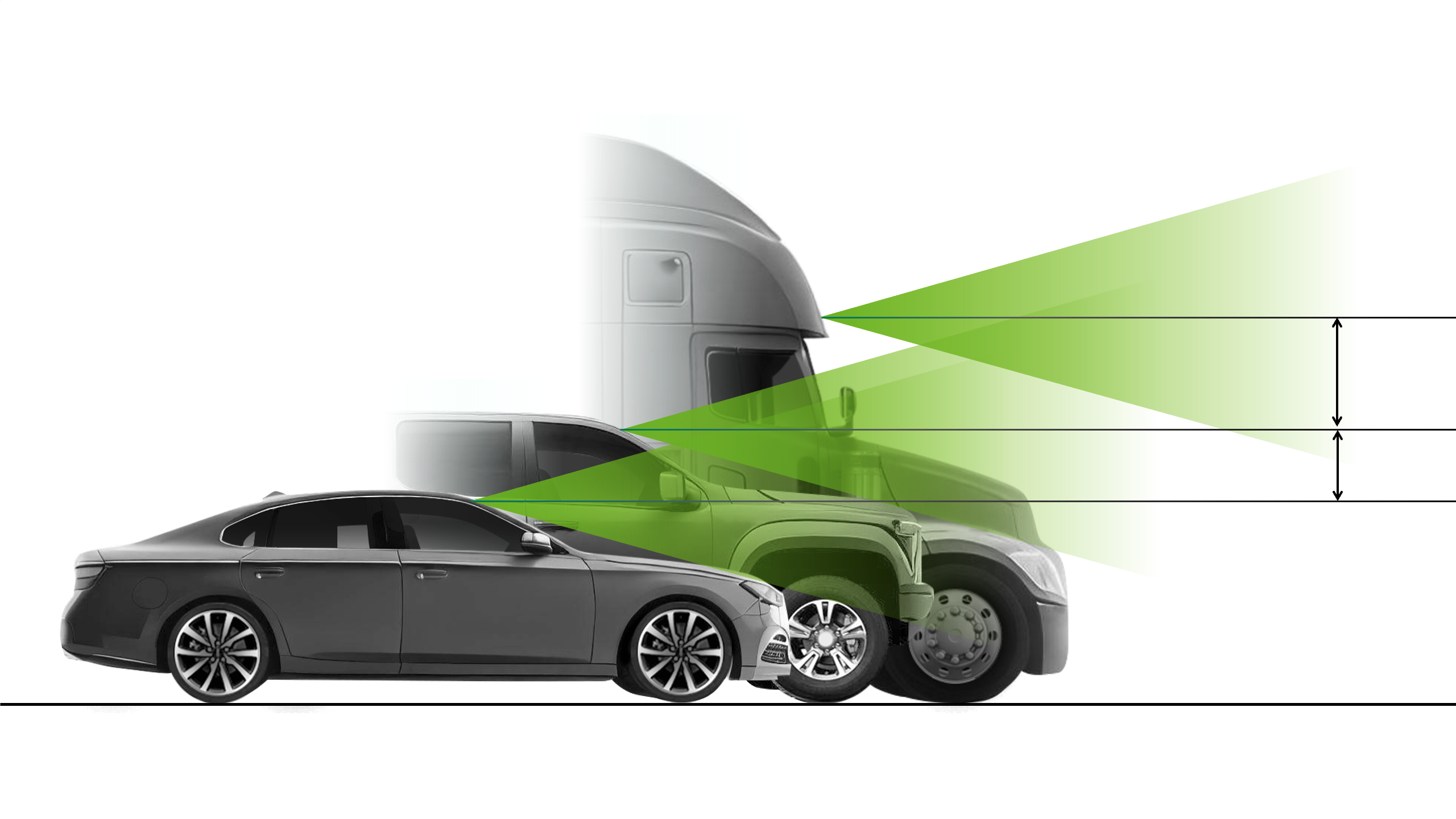
- Introduction: This blog post discusses the challenges in deploying perception algorithms on vehicles with different sensor configurations and the use of synthetic datasets and novel view synthesis (NVS) to address these challenges.
- Measuring DNN sensitivity: The first step is to create a digital twin of the test fleet vehicle in NVIDIA DRIVE Sim and vary aspects such as sensor rig height and mount position. Running inference on the generated datasets allows measuring the network's accuracy for different sensor configurations.
- Addressing perception gaps: Deploying on a new vehicle type requires a targeted dataset for viewpoints that differ from the original data. Traditional augmentations using existing fleet data are not sufficient. NVS, a computer vision method, can generate new views of a scene from existing images, allowing the creation of datasets captured from different vehicle types.
- Novel view synthesis: NVS uses lidar-based depth supervision and automasking to improve depth estimation and handle occlusions. This approach expands on prior work by generating images of a scene from different viewpoints or angles not originally captured.
- Using NVS-generated data: The NVS algorithm is validated by training a perception algorithm on a combination of fleet data and NVS-transformed data. Synthetic data and ground truth labels are generated in DRIVE Sim to test the model's performance from multiple sensor viewpoints. Results show that using NVS-generated data for training improves perception performance.